Decisions: Business Analytics — great expectations, great opportunity
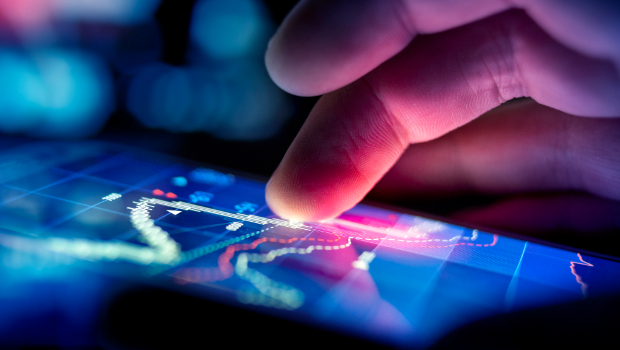
(Image: Stockfresh)
Big data and analytics tools are developing fast, as needs and resources change, but challenges remain, reports PAUL HEARNS
15 March 2019 |
Insights, intelligence, advantage — these are constant refrains when it comes to big data and analytics (BDA) tools.
The market is burgeoning, with IDC forecasting a value of €260 billion (€228.6 billion) by 2022 with a compound annual growth rate (CAGR) of 11.9% over the forecast period.
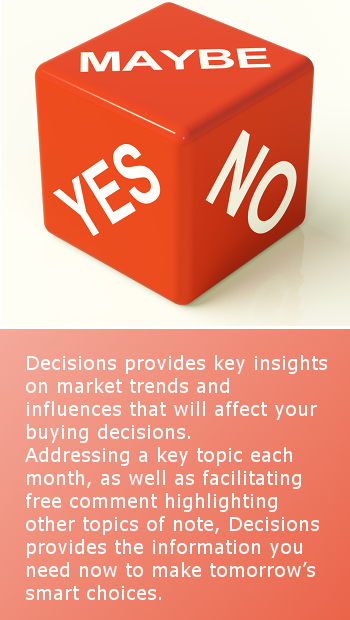
Investments are broad across industry sectors, though IDC reports banking, discrete manufacturing, process manufacturing, professional services, and federal/central government are notable verticals.
According to the analyst, these five industries will account for nearly half of worldwide BDA revenues, while also becoming the largest BDA opportunity in 2022 when their total investment will likely be $129 billion (€113.4 billion). The industries that will deliver the fastest BDA revenue growth are retail (13.5% CAGR), banking (13.2% CAGR), and professional services (12.9% CAGR), says IDC.
“At a high level, organisations are turning to Big Data and analytics solutions to navigate the convergence of their physical and digital worlds,” said Jessica Goepfert, programme vice president, Customer Insights and Analysis at IDC. “This transformation takes a different shape depending on the industry. For instance, within banking and retail – two of the fastest growth areas for Big Data and analytics – investments are all about managing and reinvigorating the customer experience. Whereas in manufacturing, firms are reinventing themselves to essentially be high tech companies, using their products as a platform to enable and deliver digital services.”
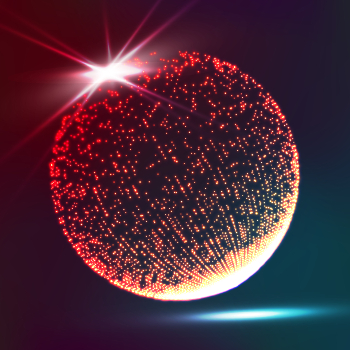
Constant research
According to Kevin Foote, writing for online resource Dataversity, many businesses, particularly those online, consider Big Data a mainstream practice. These businesses are constantly researching new tools and models to improve their Big Data utilisation.
Under such intense interest and investment, the tools are obviously evolving at a similarly accelerated rate. Foote says the continuous growth of the Internet of Things (IoT) has provided several new resources for Big Data. New technologies change not only how business intelligence is gathered, he says, but how business is done.
IoT technologies are being combined with streaming analytics and machine learning, reports Foote. Whereas ML was previously trained used stored data, now streaming sources can be employed in a similar fashion, in real time. The primary goal of which, he reports, is to provide greater flexibility and more appropriate responses to a variety of situations, with a special focus on communicating with humans.
This change from constrained training models to more open, potentially less controlled environments, requires greater complexity in algorithms to cope. The ML becomes better able to support systems in predicting outcomes with reasonable accuracy, and as the model adjusts and evolves, additional edge or cloud resources can coordinate to match changes as required.
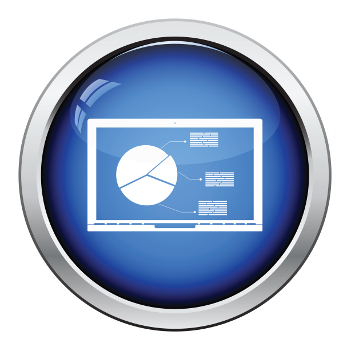
“We will see more and more businesses treat computation in terms of data flows rather than data that is just processed and landed in a database,” said Ted Dunning, chief application architect, MapR. “These data flows capture key business events and mirror business structure. A unified data fabric will be the foundation for building these large-scale flow-based systems.”
Specific architectures
Another major trend in analytics tools is for case-specific architectures for data gathering and analytics.
According to a 2018 report from Tableau, architectures are maturing to reject one-size-fits all frameworks.
“Hadoop is no longer just a batch-processing platform for data-science use cases. It has become a multi-purpose engine for ad hoc analysis. It’s even being used for operational reporting on day-to-day workloads—the kind traditionally handled by data warehouses,” says the report.
Organisations, the report states, are responding to these hybrid needs by pursuing use case-specific architecture design.
“They’ll research a host of factors including user personas, questions, volumes, frequency of access, speed of data, and level of aggregation before committing to a data strategy. These modern-reference architectures will be needs-driven.”
“They’ll combine the best self-service data-prep tools, Hadoop Core, and end-user analytics platforms in ways that can be reconfigured as those needs evolve. The flexibility of these architectures will ultimately drive technology choices,” says the report.
Data management
Despite such developments, problems still persist. Alex Woodie writing for Datanami.com, argues that data management is, and is likely to remain, hard.
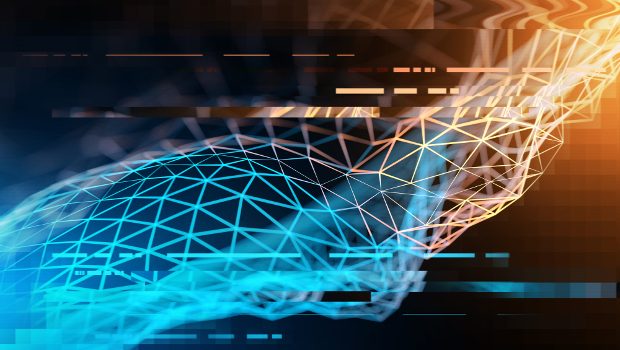
“The big idea behind big data analytics is fairly clear-cut — find interesting patterns hidden in large amounts of data, train machine learning models to spot those patterns, and implement those models into production to automatically act upon them. Rinse and repeat as necessary.”
However, he says, the reality of putting that basic recipe into production is a still harder than it looks.
“For starters, amassing data from different silos is difficult and requires extract, transform, load (ETL) and database skills. Cleaning and labelling the data for the ML training also takes a lot of time and money, particularly when deep learning techniques are used. And finally, putting such a system into production at scale in a secure and reliable fashion requires another set of skills entirely,” says Woodie.
For these reasons, he argues, data management remains a big challenge, and data engineers will continue to be among the most sought-after personas on the big data team.
Woodie also predicts that data silos will continue to proliferate, despite the efforts to unite, combine and analyse. He says that the boom in activity around Hadoop some years ago promulgated the idea of consolidation of all of our data, for both analytical and transactional workloads, onto a single platform.
“That idea never really panned out, for a variety of reasons,” he says.
“The biggest challenge is that different data types have different storage requirements. Relational database, graph databases, time-series databases, HDFS, and object stores all have their respective strengths and weakness. Developers can’t maximise strengths if they’ve crammed all their data into a one-size-fits-all data lake.”
“In some cases, amassing lots of data into a single place does make sense. Cloud data stores such as S3, for instance, are providing companies with flexible and cost-effective storage, and Hadoop continues to be a cost-effective store for unstructured data storage and analytics. But for most companies, these are simply additional silos that must be managed. They’re big and important silos, of course, but they’re not the only ones.”
In the absence of a strong centralising force, data silos will continue to proliferate, he states.
Augmented analytics
Data and the intelligence derived from it must still inform humans who must then judge how best to leverage it. That still means representing it in a way that is accessible, informative and effective.
According to Lynda Partner, VP, marketing and analytics as a service, with consultant Pythian, augmented analytic will have a transformative effect on how the insights are derived and presented.
“In 2018,” she says, “most qualitative insights are still teased out by data scientists or analysts after poring over reams of quantitative data. But with augmented data, systems use artificial intelligence and machine learning to suggest insights pre-emptively.”
Partner cites Gartner which asserts that augmented analytics will soon become a widespread feature of data preparation, management, analytics and business process management, leading to more citizen data scientists as barriers to entry come down, especially when combined with natural language processing, which makes possible interfaces that let users query their data using normal speech and phrases.
Another key trend will be for the harnessing of ‘dark data’, says Partner. Gartner calls dark data “the information assets organisations collect, process and store during regular business activities, but generally fail to use for other purposes”.
Partner argues that as organisations “increasingly leave no business intelligence-related stone unturned, we’re likely to see more emphasis placed on this as-of-yet relatively untapped resource, including the digitisation of analogue records and items (think everything from dusty old files to fossils sitting on museum shelves) and their integration into the data warehouse”.
Data storytelling
Building on this trend, data storytelling and visualisation will also see significant development.
“An increase in the use of cloud-based data integration tools and platforms means a more unified approach to data,” argues Partner, “in turn meaning more and more employees will have the ability to tell relevant, accurate stories with data using an organisation’s single version of the truth.”
“And as organisations use even better and improved integration tools to solve their data silo problems, data storytelling will become more trusted by the C-suite as insights gleaned across the organisation become more relevant to business outcomes.”
Finally, DevOps and DevSecOps are now being joined by DataOps.
According to partner, this concept emerged in 2018, but will grow significantly in importance this year, as data pipelines become more complex and require even more integration and governance tools.
“DataOps applies Agile and DevOps methods to the entire data analytics lifecycle,” Partner writes, “from collection to preparation to analysis, employing automated testing and delivery for better data quality and analytics. DataOps promotes collaboration, quality and continuous improvement, and uses statistical process control to monitor the data pipeline to ensure constant, consistent quality.”
If, as predicted, organisations should be able to handle a thousand data sources in a data warehouse, there is a need to provide automated and always-on data integration, which, Partner maintains, will be the difference between delivering value and drowning.
“Most organisations are coming to understand that their traditional data warehouse just won’t cut it. As more and more endpoints, edge devices and other data sources spur newer and newer data types, it’s imperative to stay prepared by using a flexible data platform that’s able to automate and integrate all your data sources and types at scale,” says Partner.
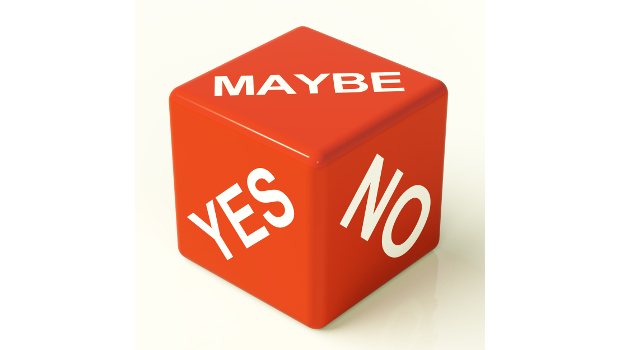
In the free comment space, Logicalis Sandra Dunne describes hybrid cloud success
Room to improve: the key to hybrid cloud success
Digital transformation is the business trend of the moment, as organisations start on a journey to using their data to drive their success or enhance their customer service. Hybrid cloud is an ideal way for organisations to achieve those goals because of how it combines long-standing on-premise IT investments with the on-demand flexibility, portability and scalability of cloud. It also meets the growing demand for business to drive IT requirements, unlike in the past where IT’s capability determined what the business could do.
If you’re expecting a ‘but’, here it comes – although it’s not what you think. The hybrid cloud model can deliver those objectives, but the problem is the way many organisations are adopting it. Some are using hybrid purely as an add-on to ‘business as usual’ mode, with some added flexibility thrown in.
Without taking the time to get the house in order from an IT perspective, the risk is that businesses with a lot of legacy infrastructure bring their bad habits with them. I’ve seen this happen before; when virtualisation first came along, it seemed like the first step on the road to delivering IT in a more agile and flexible way. But it didn’t always drive better behaviour. Just as often, the ability to spin up a virtual machine gave an easy solution to every problem, whether or not it was the right one.
Technology is a tool, and when used right it can deliver flexibility and agility that the business needs; if left unmanaged, it’s like the room in the house where you hoard everything.
In our experience, moving different applications to the cloud in whole or in part, or adopting software as a service, doesn’t take the rules away. It just defines them further.
Deciding to adopt a hybrid cloud model is an opportunity for a business to step back, look at how it does things, and make changes. First, that needs an understanding of the organisation’s current state. Without this crucial stage, it’s like building an extension to your house just to contain all the stuff you’ve accumulated over years.
If we think of our IT infrastructure as a house we’ve built, then the first step in moving to a hybrid cloud model is a thorough spring cleaning. It’s the chance to redefine policies around how long the business retains data; in some cases, it’s an ideal time to redefine the IT strategy outright.
IT leaders need to manage their resources in the hybrid cloud world just as carefully as when they were on-premise. It carries the same responsibilities from a data protection perspective. What’s more, doing things the same way means that the cost could be higher with hybrid than with traditional in-house IT. That’s because the cloud’s pay-as-you-go model means that any inefficient storage or server capacity will cost more. That’s not what the cloud promised, but it’s what could easily happen.
Adopting a hybrid cloud model is an opportunity to press the reset button; to do something new and different, as opposed to doing the same thing in a different place.
Sandra Dunne, data centre solutions manager, Logicalis
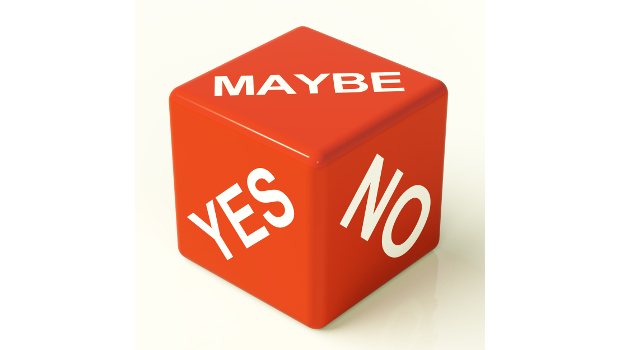