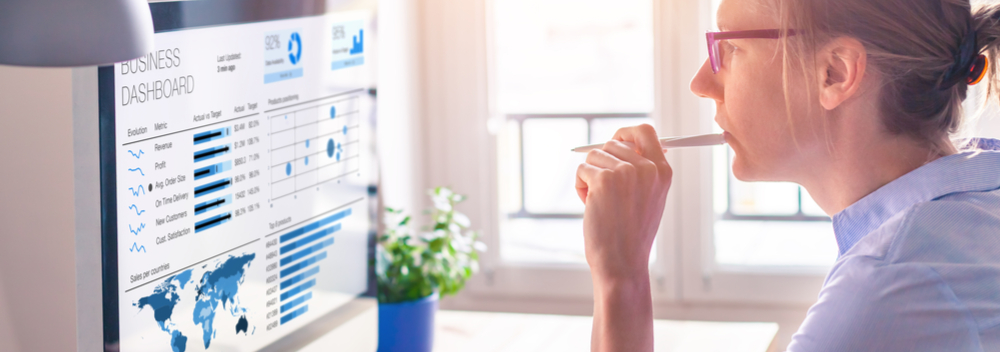
As companies start or continue on their digital transformation journey, the amount of data they deal with grows in volume and complexity.
Traditional analytics — the process of preparing and analyzing data, interpreting results and telling stories with that data — is a manual, time-intensive process. And it poses significant challenges for companies that are trying to deal with the continuous burst of data.
In order to meet digital transformation market-readiness timelines, it has become imperative to reduce the time-intensiveness of manual analytics.
To do this, we can augment human data scientists with machine learning (ML) and artificial intelligence (AI) to speed up each step in the analytics journey – something called augmented analytics.
For the past 12-18 months, augmented analytics as a field has grown and more enterprises are beginning to accept this process.
Companies undergoing digital transformation across many industries such as pharmaceutical, retail, manufacturing and more are beginning to put augmented analytics to use.
I believe that in the coming year this emerging field will become more broadly used and understood.
Brief background on augmented analytics
In the mid-2000s, cloud storage and massively available computing power became more mainstream. This caused big data analytics to explode.
This was a significant change, but the process was still highly manual – meaning analytics professionals still had to write volumes of code.
Before the big data revolution, companies might have needed to scan 1000s of flows to gain meaningful insights, but today we’re talking about millions of flows.
The question has become: how can data analytics deliver the same value while tremendously reducing the time required, knowing that faster analytics = faster time to market = stronger ROI.
This is what augmented analytics is intended for — speeding up the field of data analytics to derive more value
As the need to wrangle and understand growing volumes of data exists, augmented analytics represents a major shift in this field of study.
Augmented analytics is already causing disruption to the interpretation, creation and sharing of analytics. In turn, this enables data scientists to spend less time exploring data, and more time acting on the most relevant insights than with traditional, manual methods.
This approach is a powerful mechanism that enables companies to be more responsive and agile – a necessary aspect of digital transformation.
Let’s first look at the key benefits of augmented analytics and how this practice is changing traditional business models. Augmented analytics:
- accelerates the data preparation and discovery process;
- democratizes data analytics for less business-savvy users;
- enables adoption of actionable insights for the executive team and across the entire organization.
The importance of these benefits to digital businesses is significant
It’s well understood that for organizations to compete in the Digital Age, data is key to gaining relevant, actionable insights.
Yet the underlying complexities inherent in manual analytics processes create many roadblocks.
For one thing, data scientists who are skilled at the practice of data science and who also possess a keen understanding of business models and operations are rare.
Additionally, a data scientist’s time is valuable — yet a large percentage of this time is spent manually preparing data through cleaning and labeling. This shortage of time and capacity means that most data analytics are performed on a small portion of the data, while a large portion of data assets aren’t mined.
Let’s explore the benefits listed above to see how truly disruptive augmented analytics are.
Three main benefits of augmented analytics
1. Accelerates data preparation and discovery
When performed manually, data preparation is a cumbersome, complex effort.
When data scientists have millions of records to comb through, a presumably simple task of finding all customers from a particular region, for example, can literally take months.
Take the example of a large specialty paper products retailer. As part of their digital journey, they needed to understand customers better, but data coming from legacy systems had poor quality.
Not only were there huge volumes of data to examine, but there were inconsistencies in various fields, and reconciling them into one unified format was daunting.
There were 26 million records to assess, in 1200 different XML file formats.
To clean the data with manual processes would have taken six to 12 months. By utilizing augmented analytics data preparation techniques and ML algorithms, the retailer was able to clean the data in about three weeks.
By automating these iterative steps, the entire data preparation and discovery time can be shortened by 50-80%.
Imagine how much more productive and efficient your data analytics team could be if this were the case!
2. Democratizes data analytics
After the data is prepared, augmented analytics detects signals that impact business models that less business-savvy data scientists may not be adept at finding.
With augmented analytics, data scientists don’t have to determine the appropriate algorithm to use or write code to get results.
An augmented analytics toolset will run eight to 10 algorithms on the data and fit it into a common format to detect patterns and outliers.
For example, a large enterprise may want insights into invoicing from their vendors to determine if there are any outliers that warrant more investigation.
With operations and employees around the globe, this can equal bills coming in from thousands or hundreds of thousands of vendors every month.
Based on the underlying data, augmented analytics will start detecting patterns and automatically create an outlier analysis to detect when a particular vendor, which consistently invoices the company $50,000 per month, sends an invoice for $500,000.
While this wouldn’t be automatically flagged as fraudulent activity, the larger-than-usual amount would be flagged as something for the data analytics professional to investigate further, and removes the burden of first writing the algorithm to make this discovery.
In other words, augmented analytics democratizes the insights, making it easy for business users to extract complex insights and saving them significant time in doing so.
3. Enables adoption of actionable insights for executives and across an organization
Once signals and patterns have been determined, the results need to be communicated with executives.
Traditional ways of doing this, such as creating a report or dashboard, puts additional burdens on executives who typically don’t have time to log into a platform and do their own data interpretation.
With augmented analytics, the tool will read the chart or report and translate the information into natural language statements such as “Your sales are decreasing in region X, a trend that has been ongoing over the past number of months.” Or, “You are losing market share to competitor Y.”
Team leaders can get answers to questions such as “sales have increased 10% at a broad level, but how are margins at each regional level?” Providing this level of actionable insights enables data-driven decision making and increases adoption across the entire organization.
Sophisticated analytics systems offer voice and natural language processing capabilities, and are embedded into enterprise search interfaces and BI platforms.
Imagine this: data scientists can interact with augmented analytics platforms using natural language simply by asking a question, such as: “how do our sales efforts compare with our competitors?”
Augmented analytics addresses behind-the-scenes complexities making the data analytics process simple for business users and citizen data scientists alike.
How can you move forward with augmented analytics?
With the benefits outlined above and the technologies available that enable this major disruption, the question is not whether you should adopt augmented analytics. The question is when to start, and how.
While there are several types of tools for the different steps discussed above, there is no one tool that performs all functions or fits all organizations.
The best way for companies to proceed is to work with a consulting partner that has broad experience in analytics and in emerging augmented analytics toolsets and methodologies to create a custom roadmap. To create a successful path forward, the right combination of people, processes and technology need to come together.
Augmented analytics is still an evolving field. Today, most companies are not adopting augmented analytics for the entire end-to-end process but are starting with one small piece – which is a good way to go.
In the next few years, I expect that that will change, and organizations will be using augmented analytics for the entire data analytics lifecycle.
In 2019, the significant benefits that augmented analytics provide are the key areas to consider: speed, democratization and broad adoption.
With these capabilities, organizations are well-equipped to understand and anticipate customer needs, adjust and improve business processes and position themselves for success in – today, and well into the future.